Anthropic cracks open the black box to see how AI comes up with the stuff it says
2 min read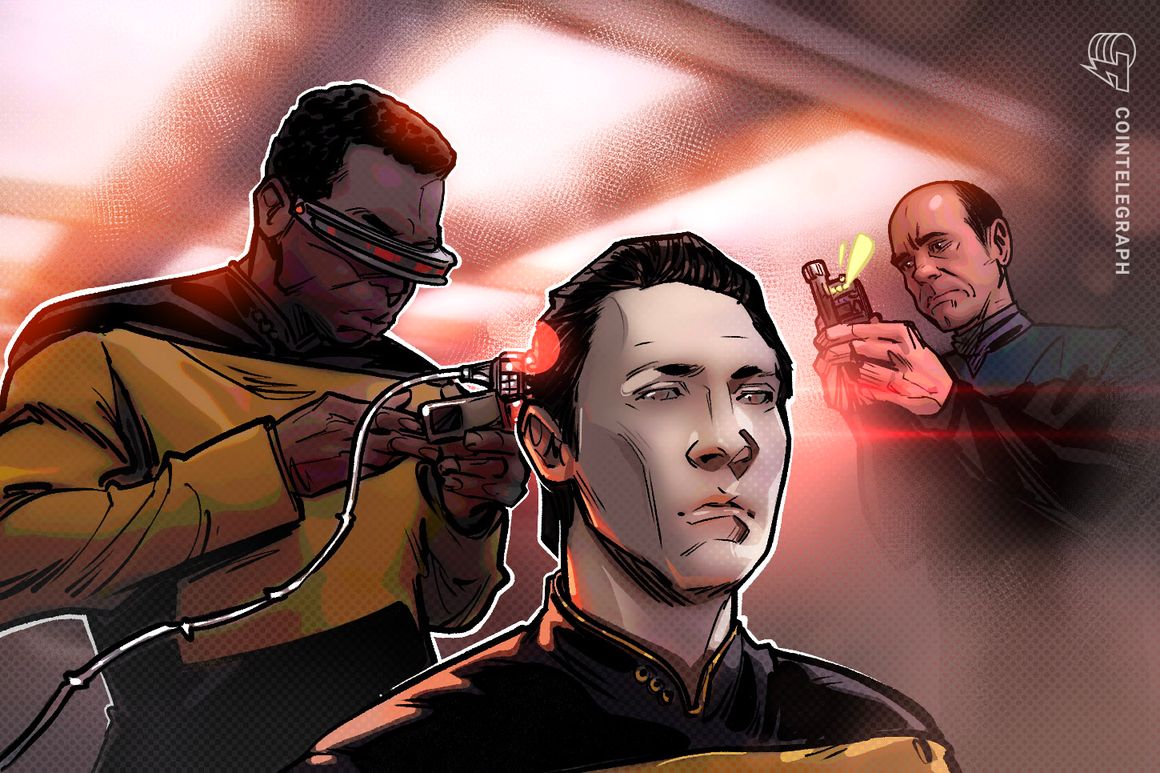
Anthropic, the artificial intelligence (AI) research organization responsible for the Claude large language model (LLM), recently
If the models were purely beholden to their training data, researchers would imagine that the same model would always answer the same prompt with identical text. However, it’s widely reported that users giving specific models the exact same prompts have experienced variability in the outputs.
But an AI’s outputs can’t really be traced directly to their inputs because the “surface” of the AI, the layer where outputs are generated, is just one of many different layers where data is processed. Making the challenge harder is that there’s no indication that a model uses the same neurons or pathways to process separate queries, even if those queries are the same.
So, instead of solely trying to trace neural pathways backward from each individual output, Anthropic combined pathway analysis with a deep statistical and probability analysis called “influence functions” to see how the different layers typically interacted with data as prompts entered the system.
This somewhat forensic approach relies on complex calculations and broad analysis of the models. However, its results indicate that the models tested — which ranged in sizes equivalent to the average open source LLM all the way up to massive models — don’t rely on rote memorization of training data to generate outputs.
This work is just the beginning. We hope to analyze the interactions between pretraining and finetuning, and combine influence functions with mechanistic interpretability to reverse engineer the associated circuits. You can read more on our blog: https://t.co/sZ3e0Ud3en
— Anthropic (@AnthropicAI) August 8, 2023
The confluence of neural network layers along with the massive size of the datasets means the scope of this current research is limited to pre-trained models that haven’t been fine-tuned. Its results aren’t quite applicable to Claude 2 or GPT-4 yet, but this research appears to be a stepping stone in that direction.
Going forward, the team hopes to apply these techniques to more sophisticated models and, eventually, to develop a method for determining exactly what each neuron in a neural network is doing as a model functions.